Of Data, Devils, and Details
A recent preprint study has plenty of gremlins in its data, perhaps enough to invalidate the conclusions
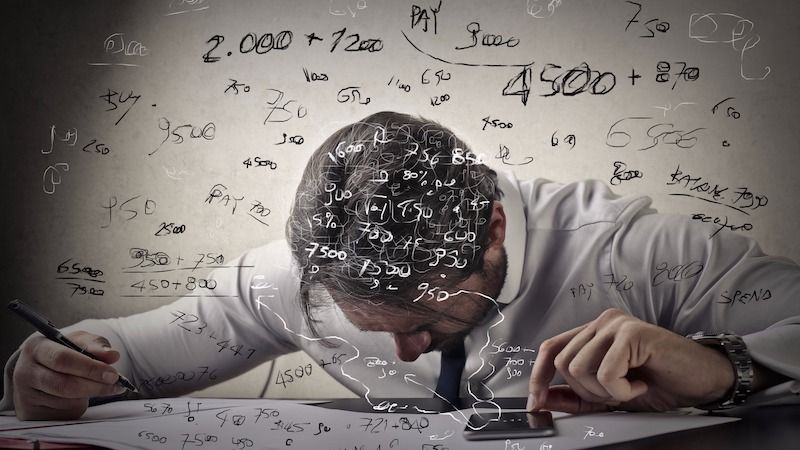
Are you ready for some post-publication review?
A couple of weeks ago, I wrote about two studies touting preprints as reliable, both of which seemed a little bizarre while exhibiting some publication problems of their own.
The first paper touting preprints as reliable, published in BMJ Medicine, didn’t include the article’s customary Review History. The editorial team is still trying to retrieve this, weeks later. Then, when I requested data from the authors via email, they claimed to have relied solely on a data source not listed in the paper.
The editor has been informed of the discrepancy in methods reporting.
The second paper touting preprints as reliable, in The Lancet: Global Health, didn’t include the list of articles covered, except in a table without enough detail to allow any secondary analyses. I requested the data from the corresponding author, got a bit of a run-around about social justice-like issues, finally complained to the editor, and last week received from the corresponding author a list of PMIDs for the articles included in the study.
Using the PMIDs to look at various publication events for these preprint-paper pairs, I found some data discrepancies, and a fundamental flaw — one which I consistently find with such analyses (see Twitter feed, below, for evidence that this is a consistent and predictable flaw).
First, some discrepancies.
- Not 100 articles, but 96. The authors claim in the paper to have analyzed “100 matched preprint–journal-article pairs using the NIH iSearch COVID-19 Portfolio,” yet the dataset shared includes only 96 articles. That’s a 4% difference — a small but notable discrepancy, especially as it the primary measure of the population (i.e., how many are in it).
- The NIH iSearch COVID-19 Portfolio Has Inconsistent Coverage. The authors claim their data source covers preprint “servers arXiv, bioRxiv, ChemRxiv, medRxiv, Preprints.org, Qeios, and Research Square.” Yet, in three cases, duplicate preprints were not identified. One was on arXiv as well as medRxiv, and two were on SSRN (Preprints at the Lancet) and medRxiv. In all three cases, the earliest preprint was not the one listed in the Portfolio, another problem if you’re measuring difference from a presumed first draft. In one other case, the Portfolio had no preprint listed for a paper despite including the supposed preprint-paper match in the analysis. I found the preprint — it is on medRxiv, and there are four versions of it.
- Publication dates in the NIH iSearch COVID-19 Portfolio Appear to Be Generally Incorrect. Perhaps due to data processing lag at PubMed, or pulling from the wrong field, or some combination, the dates of journal publication in the Portfolio were generally wrong whenever I checked them. This doesn’t bear directly on the paper, but it does raise another question about QA around the dataset overall.
- Preprints Aren’t Always Papers. A good number of preprints ended up as Letters to the Editor or Correspondence in the publishing journal, an editorial designation that usually means the findings are either rather narrow or a bit far afield. Preprint servers don’t capture this subtle journal culture distinction, and it’s worth asking if preprints that end up at LTEs deserve to be in this analysis.
- 1/3 of the Preprints Included Had More Than One Version. Of the 96 preprint-paper pairs, 33 preprints had more than one version posted — in six cases, four or more versions were posted. Yet, the authors aren’t clear which version was used for comparison.
Now, for the problem that might undercut the entire premise of the paper, which as you might recall asserts that there is little meaningful difference between the preprint and the published work when it comes to the underlying data, which the authors generalize to mean that preprinting in the wild is safe and reliable enough.
In their comparisons, they specifically bin preprints as existing “before peer review” and papers as existing “after peer review.”
Generally, there is no way of knowing whether a preprint is a version of a paper that has never undergone peer review. Many authors are cautious, and only post preprints once they know they have found a journal where their paper is likely to be accepted. Many authors start submissions at their field’s most-respected journal, and many of these journals provide peer-review prior to rejection. Therefore, an unknowable amount of peer-review may be occurring before any preprint is posted.
Now to the specifics in this paper. As it turns out, the majority of the papers in the set (54 of 96) were posted as preprints 5.5 days after they were submitted to journals, with many posted weeks later. This comports with the cautiousness of authors. In addition, there are multiple reasons to believe many were posted after peer-review:
- 17 (17.7%) were posted as a preprint more than 2 weeks after submission
- 12 (12.5%) were posted more than a month after submission
- 19 (19.7%) were posted as preprints after acceptance
- 5 (5.2%) were posted as preprints on or after the publication date of their peer-reviewed article
Most people studying preprinting fail to take into account the dates associated with preprinting, journal submission, and editorial acceptance. The database used here doesn’t include such publication event dates. Yet, in this paper, more than 1 in 5 preprints were posted after they’d been peer-reviewed, editorially reviewed, and accepted by a journal.
Clearly, this paper can’t claim to have separated the effects of peer-review out of the papers selected for this study.
I’ve shared these findings with the corresponding author and the editor of The Lancet: Global Health. I will also be submitting a letter to the journal to allow a formal response.
It’s a little astounding to me that peer-reviewers and editors can be so credulous about claims that their work doesn’t make a difference. Some form of ideological capture continues to shape our attitudes about epistemology — and it’s corrosive.
A final note — this came after another set of studies presented at the Peer-Review Congress in September claimed similar findings. In a Twitter exchange with a presenter and a defender of such claims, I noted that this very confounder is an often-overlooked pitfall for such studies:
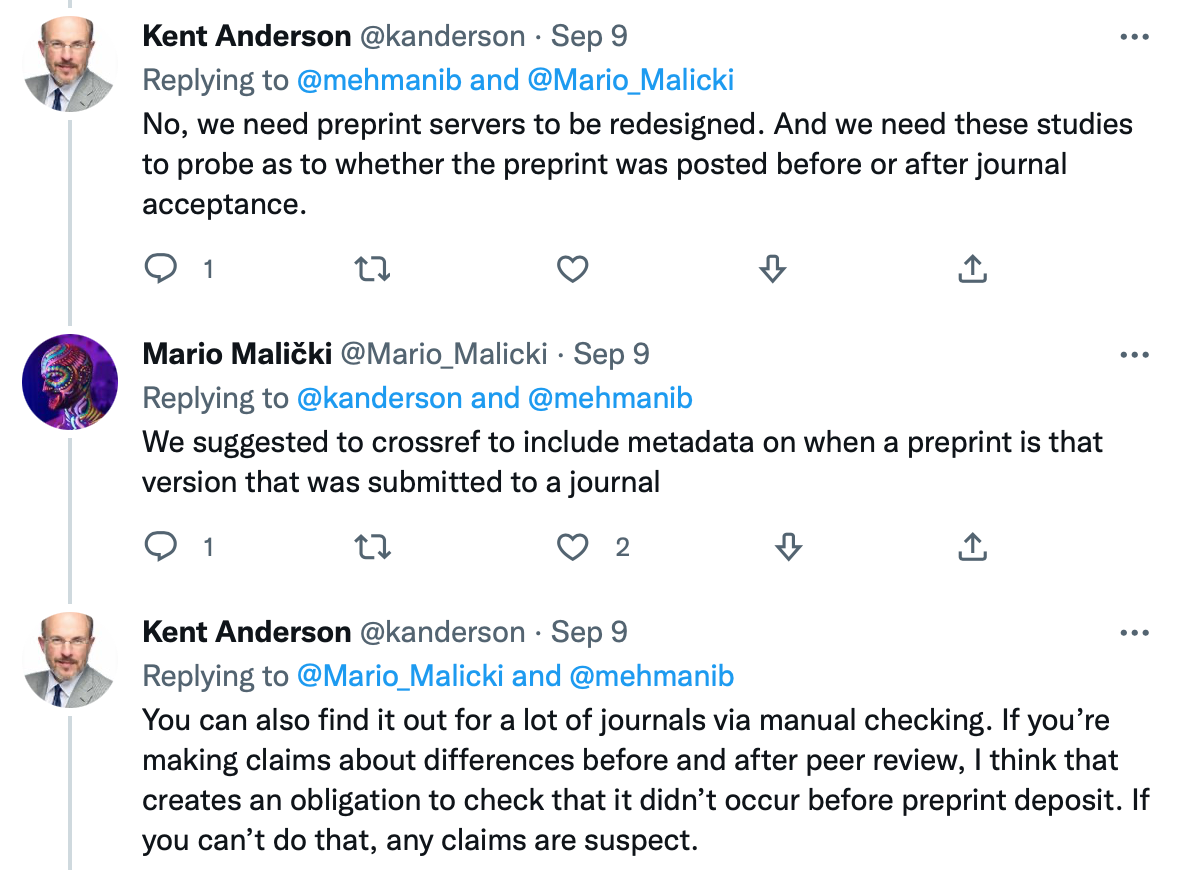
Studying data requires healthy skepticism, accurate data that measures every relevant major variable, and objectivity about what it may or may not show. These studies so far don’t address major confounders, don’t have accurate or complete data, and fail to prove their hypotheses.
Onward.