Flawed Data, Bad Assumptions
Again and again, preprint analyses fail to account for the amount of peer-review occurring before preprints are posted
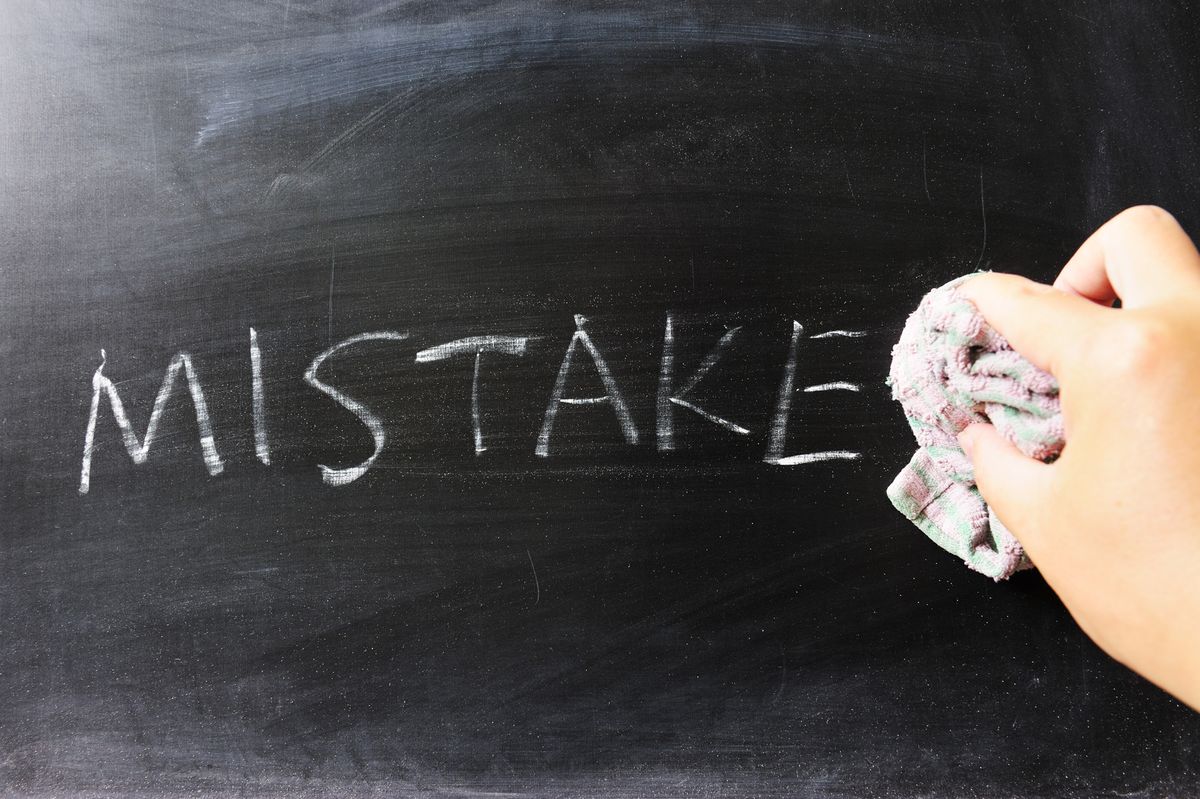
Just before the end of the year, I wrote about three flawed preprint studies — one in BMJ Medicine, one in Lancet: Global Health, and a new one at the time in JAMA Network Open.
I’d gone into some detail about the first two in prior posts.
This post details problems with the JAMA Network Open study, which stem from bad assumptions that led to incomplete data, which was then mismanaged.
The study’s authors define preprints as “preliminary research reports that have not yet undergone peer review.” Given a great deal of documentation that this is not the case — AAM’s are becoming a greater share of preprint repositories with each passing year — this definition’s simplicity signaled a flaw in the baseline assumptions.
The authors’ goal was to measure concordance between preprints and final published works by comparing the data and conclusions. They claim to have found a high level of concordance, and suggest this means preprints are reliable prior to journal review, and by extension that journal review changes preprints little.
For this to be a strong claim, the authors would have to know that the preprints they studied were not reviewed by a journal prior to being posted.
They did not measure for this, a classic red flag.
Interactions with an editorial office, peer reviewers, or some combination of the two prior to a preprint being posted could muddy any comparison of preprints and papers. If a good percentage of the preprints in the study were posted after many weeks of journal interaction — worse, after journal acceptance — a lot of questions about the data and any related conclusions would naturally arise.
Are you sitting down?
Of the nearly 1,400 papers in the study, 644 are shown in the data to have been published in peer-reviewed journals as of the study cutoff date. (Hold onto that “644” number — it will come in handy when we talk about data availability.)
Of these, 31.8% of the preprints were posted 2 or more days after journal submission, 20.5% were posted 10 or more days after journal submission, 14.3% were posted 20 or more days after journal submission, and 3.1% were posted after acceptance.
On average, preprints posted after submission were posted 38 days later. The longest span of time was 441 days, the minimum 2 days (I excluded 1-day differences).
This many preprints posted after meaningful editorial office interactions, peer-review, or both, could well explain a high proportion of the observed concordance between versions — because they were essentially peer-reviewed manuscripts posted as preprints.
The “before” is not exactly what the authors seem to have assumed.
Data availability problems
The authors stated in their December 9, 2022, paper that data would be available upon publication via an Excel spreadsheet. I emailed the authors on December 29 as instructed in their data availability statement, and received a note about a week later (and after a second request) telling me that they were still working on a user-friendly version of the data.
This means they did not meet their obligation for when the data would be available, and they created a different set of data from what they actually used in the study.
How different? In the study, they counted “547 clinical studies that were initially posted to medRxiv and later published in peer-reviewed journals.” Yet, the data they have posted has publication DOI links for 644 clinical studies within the same 1,399 preprint population. That’s nearly 100 more.
Their user-friendly version of the data does not appear to be the same data used for the study. It’s impossible to know what other revisions or changes were made, and there’s no way of knowing the subset of the 644 that was used for the 547 mentioned in the study.
This is just the most obvious difference to be found. Were there fields excluded? New ones added?
It’s worth remembering that the authors of the Lancet: Global Health study also had totals in the text that diverged from the totals ultimately shown in their data.
Researchers aren’t great data curators, as evidenced here. In addition to the egregious problem of generating new data without realizing it because you generated it later than promised, within most datasets, there are issues — in this case, a few bad DOIs, a few dead links. Worse, the overall conception of the dataset is inadequate, requiring the user to supplement it because the study was started with the flawed assumption that preprints are always posted prior to peer-review.
(We can also recall that the researchers publishing in BMJ Medicine didn’t even use the data sources they claimed in the paper.)
I mentioned earlier this week that I don’t trust authors — especially when a chatbot has been given authorship status. But even for humans, this helps explain why. They latch onto a narrative, and can become blinkered. It’s human nature.
Am I right about the flaws here? I don’t know, but I think there’s more nuance and complexity when you widen the lens beyond the initial, oversimplified assumptions and the resulting limits on the dataset.
These three papers may share a set of problems that deserve a more serious examination. Publishing definitely deserves more serious and competent researchers. It might be time to pull together an analysis of what went wrong with these three, once more of the story becomes available.