Whiplash — and Data in Medicine
PLOS' new journal needs to pull back on the hype, but early indications are it won't
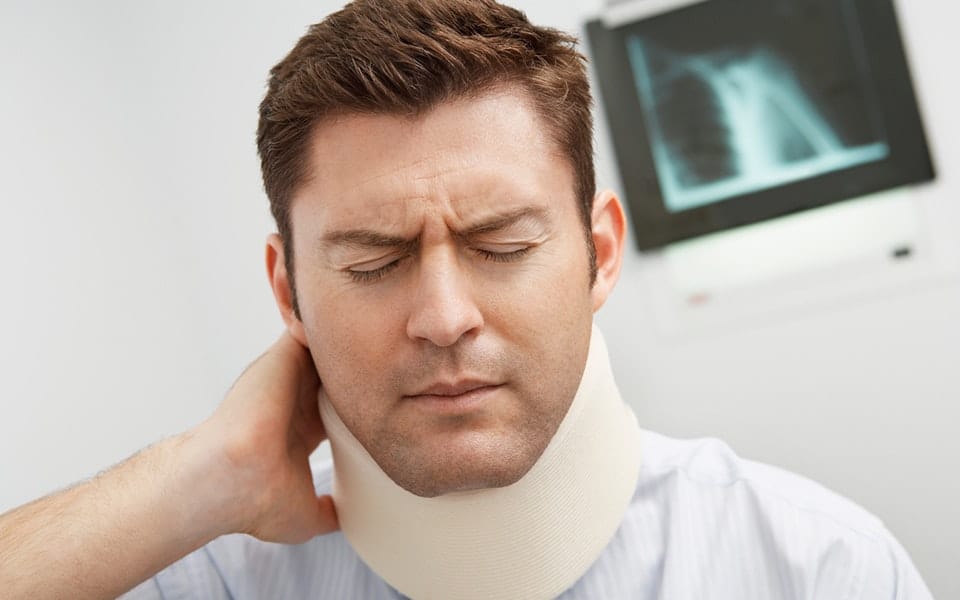
The Boston Globe made my head spin a little in the last few days, publishing a thoughtful analysis syndicated from STAT News about AI’s credibility crisis in medicine in which all the Covid-19 papers touting machine learning models to help tackle the pandemic proved to be fatally flawed, then following it today with a puff piece — also from STAT News — about PLOS’ new journal, PLOS Digital Health.
The Globe proved it’s a sloppy user of syndicated content. Both of these articles appeared in the Boston Globe within 24 hours of each other — the “credibility crisis” article in the Sunday edition, the puff piece in today’s. However, the article about PLOS Digital Health was originally published by STAT News on May 5, while the credibility crisis article was published June 2. So, the editorial team at the Boston Globe earned some criticism for not being more timely or coordinating coverage in a more considered manner.
But there are more important issues to consider when the coverages are contrasted.
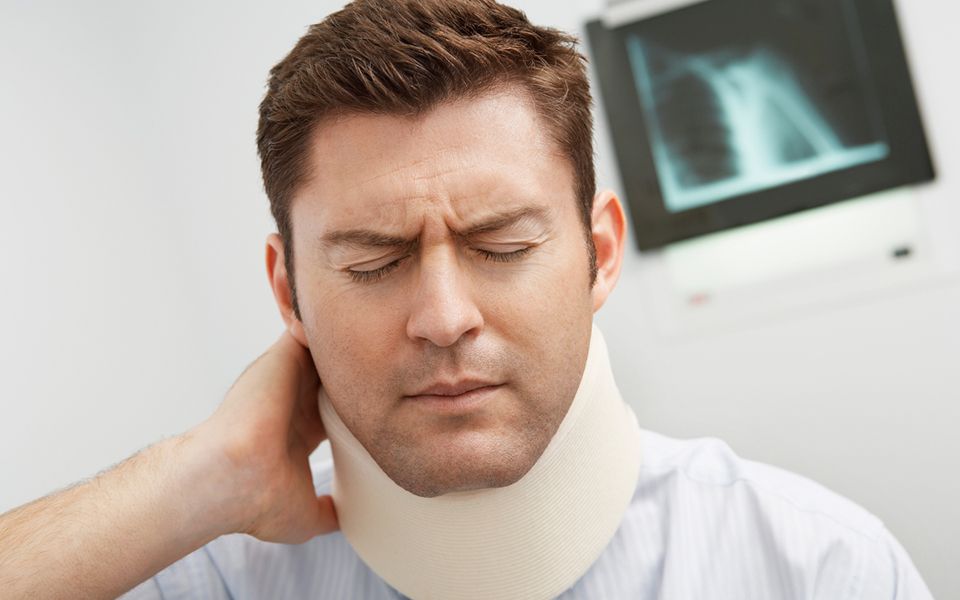
The puff piece is an interview with the EIC of PLOS Digital Health, and consists of all the usual bon mots of data science and open access — an ill-defined call for democratized data, a downplaying of politics and economics as if they can just be wished away, and silly statements like “[algorithms] accuracy is bound by space and time.” You know, space . . . and time — things that always come to mind when I think of algorithms.
Meanwhile, the critique of AI and machine learning in medicine is far more thoughtful and realistic, citing a March study from the University of Cambridge of more than 400 papers touting AI and machine learning approaches to Covid-19 which found that every single one was fatally flawed.
Calling this a “polluted area of research,” one author believes these problems have sown a deeper distrust in the field than existed before Covid-19 and these unvalidated papers, many of which were distributed and promoted via preprint servers. As the author of the STAT News piece writes:
The review . . . found that many studies not only lacked external validation but also neglected to specify the data sources used or provide details on how their AI models were trained. All but 62 of the more than 400 papers failed to pass an initial quality screening because of these omissions and other lapses.
That’s about a 15% rate for passing the initial screening — lower than the rule of thumb for a biomedical preprint reaching publication (~30%), which itself may have been lowered by the flood of low-quality, hastily assembled Covid-19 research in data science and otherwise.
But it got worse from there:
Even the studies that survived the initial screening suffered from multiple shortcoming — 55 of those 62 papers were found to be at high risk of bias due to a variety of problems, including reliance on public data sets where many imaged suspected to represent Covid-19 were not confirmed to be positive cases.
Working through the publication events here, the Boston Globe made the biggest mistake in my opinion — following a fair and damning analysis of the problems with AI and machine learning in medicine with a puff piece about a new PLOS journal, even though the puff piece was published initially a month earlier.
The bottom line is that data science has a long way to go before it can be trusted in medicine, while the hype over AI is causing some subtle damage I don’t hear discussed much — that is, it’s having a chilling effect on the field overall, as people brace for what is being sold as an inevitable medical future full of machines and devoid of humans. I was talking with a radiologist friend this weekend, and one of the effects of all this hype about AI and machine learning disrupting various medical fields has been that trainees have been driven out — they’ve internalized the notion that any career in radiology, epidemiology, neurology (well, nearly all “-ologies”) will be short-lived once the machines get involved. The truth has been otherwise — the limits of AI are glaring, the need for humans manifest, and the mythology of AI harmful in ways we might have anticipated if we’d been a bit more measured in our approaches to the future.
Are we ready to pull back on the hype now? We’ll see if PLOS Digital Health will be a source of more heat than light. Let’s hope they have the willpower to resist the temptations to cheerlead AI and machine learning, and to scrutinize the evidence carefully and stop driving a mythological narrative.